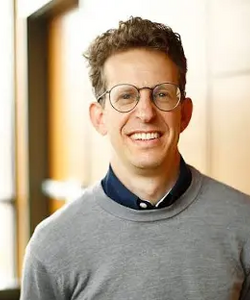
Bohrer Lecture: Dylan Small - Wharton, University of Pennsylvania
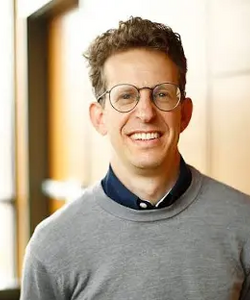
Talk Title: Exploration, Confirmation and Replication in the Same Observational Study
Talk Abstract: Exploratory data analysis, confirmatory data analysis and replication are three important aspects of building strong evidence from observational studies. Exploratory data analysis, confirmatory data analysis and replication are often thought of as being done on separate studies. However, for settings where randomized experiments are impossible to conduct for ethical reasons and observational studies must be relied on, it is common that there is a data set with unique strengths. We develop a two-team cross screening approach that allows for exploratory data analysis, confirmatory data analysis and replication to be done in the same observational study data set. We apply the approach to study the effect of unwanted pregnancy on mothers' later life outcomes using data from the Wisconsin Longitudinal Study. We will also briefly discuss data turnover, a one team cross-screening approach that provides some of the benefits of two-team cross screening with only one team. This is joint work with Samrat Roy, Marina Bogomolov, Ruth Heller, Amy Claridge, Tishra Beeson and Will Bekerman.
Wijsman Lecture: Ming Yuan - Columbia University
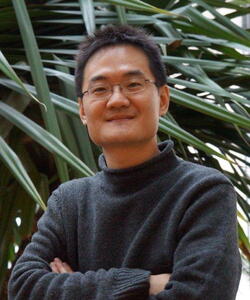
Talk Title: : A cumulant approach to linear regression
Talk Abstract: Linear regression is a cornerstone of statistics. Despite its generality and flexibility, its interpretation can be subtle. In this talk I will describe a new perspective to linear regression through the lens of higher order cumulants and discuss how it offers new insights into a number of classical problems including the omitted variable bias.
Past Bohrer Workshop Keynote Speakers
- 1994 Mark Schervish, Carnegie Mellon University
- 1995 Dan Naiman, Johns Hopkins University
- 1997 Ross Leadbetter, University of North Carolina
- 1998 Dennis Karney, University of Kansas
- 1999 Erich Lehmann, University of California Berkeley
- 2000 David Bartholomew, London School of Economics
- 2001 Gary Koch, University of North Carolina
- 2002 Robert Serfling, University of Texas Arlington
- 2003 Peter Bickel, University of California Berkeley
- 2004 Peter Imrey, Cleveland Clinic Foundation
- 2005 John Marden, University of Illinois
- 2006 Raymond Carroll, Texas A&M University
- 2007 Mary Ellen Bock, Purdue University
- 2008 Ker-Chau Li, UCLA
- 2010 Zhiliang Ying, Columbia University
- 2011 Minge Xie, Rutgers University
- 2012 Xuming He, University of Michigan
- 2013 Yuhong Yang, University of Minnesota
- Sky Andrecheck, Cleveland Indians
- 2014 Hua-Hua Chang, University of Illinois at Urbana-Champaign
- 2015 Cun-Hui Zhang, Rutgers University
- 2016 Lawrence Brown, University of Pennsylvania
- 2017 Edward George, University of Pennsylvania
- 2018 Regina Liu, Rutgers University
- 2019 - 2020 Cancelled
- 2021 Liza Levina, University of Michigan
- 2022 Vijay Nair, University of Michigan
- 2023 Andrew R. Barron, Yale University
- 2024 Huixia Judy Wang, George Washington University
Past Wijsman Lecturers
- 2014 Zhiliang Ying, Columbia University
- 2015 John Lafferty, University of Chicago
- 2016 Xihong Lin, Harvard University
- 2017 Nancy Reid, University of Toronto
- 2018 Michael Kosorok, University of North Carolina at Chapel Hill
- 2019 - 2020 Cancelled
- 2021 Dean Foster, Amazon
- 2022 Dawn Woodard, Uber
- 2023 Jelena Bradic, University of California San Diego
- 2024 Bodhisattva Sen, Columbia University